Will AI help or hinder trust in science?
Google DeepMinds AlphaFold model has revolutionised how scientists predict protein structures.But there are many other, less well publicised, successes too such as AI being used at CSIRO to discover new galaxies in the night sky, IBM Research developing AI that rediscovered Keplers third law of planetary motion, or Samsung AI building AI that was able to reproduce Nobel prize winning scientific breakthroughs.When it comes to narrow AI applied to science, trust remains high.AI systems especially those based on machine learning methods rarely achieve 100 per cent accuracy on a given task.
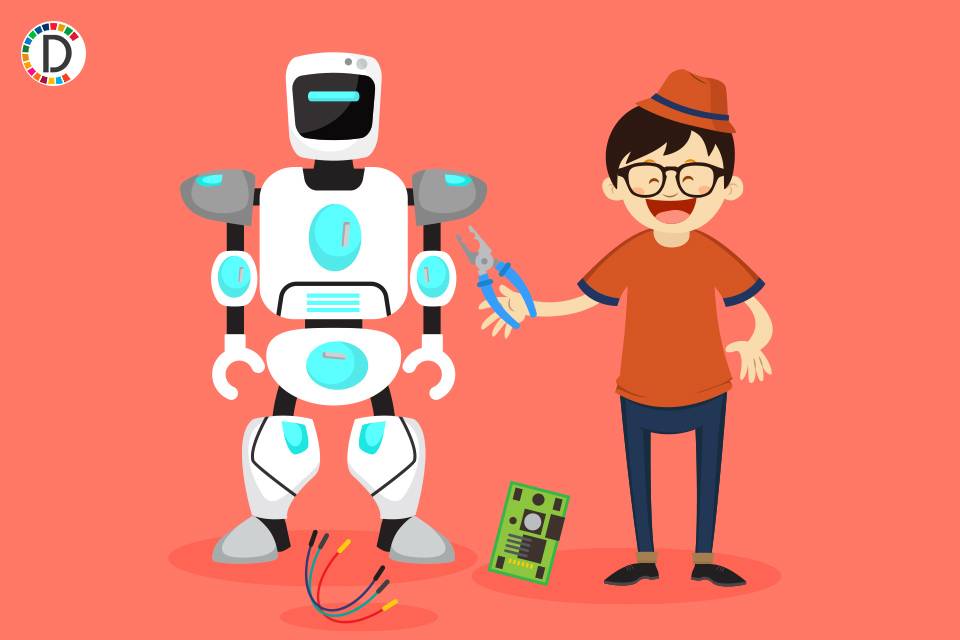
- Country:
- Australia
Melbourne, Apr 15 (360info) In the past year, generative artificial intelligence tools — such as ChatGPT, Gemini, and OpenAI's video generation tool Sora — have captured the public's imagination.
All that is needed to start experimenting with AI is an internet connection and a web browser. You can interact with AI like you would with a human assistant: by talking to it, writing to it, showing it images or videos, or all of the above.
While this capability marks entirely new terrain for the general public, scientists have used AI as a tool for many years.
But with greater public knowledge of AI will come greater public scrutiny of how it's being used by scientists.
AI is already revolutionising science — six per cent of all scientific work leverages AI, not just in computer science, but in chemistry, physics, psychology and environmental science.
Nature, one of the world's most prestigious scientific journals, included ChatGPT on its 2023 Nature's 10 list of the world's most influential and, until then, exclusively human scientists.
The use of AI in science is twofold.
At one level, AI can make scientists more productive.
When Google DeepMind released an AI-generated dataset of more than 380,000 novel material compounds, Lawrence Berkeley Lab used AI to run compound synthesis experiments at a scale orders of magnitude larger than what could be accomplished by humans.
But AI has even greater potential: to enable scientists to make discoveries that otherwise would not be possible at all.
It was an AI algorithm that for the first time found signal patterns in brain-activity data that pointed to the onset of epileptic seizures, a feat that not even the most experienced human neurologist can repeat.
Early success stories of the use of AI in science have led some to imagine a future in which scientists will collaborate with AI scientific assistants as part of their daily work.
That future is already here. CSIRO researchers are experimenting with AI science agents and have developed robots that can follow spoken language instructions to carry out scientific tasks during fieldwork.
While modern AI systems are impressively powerful — especially so-called artificial general intelligence tools such as ChatGPT and Gemini — they also have drawbacks.
Generative AI systems are susceptible to ''hallucinations'' where they make up facts.
Or they can be biased. Google's Gemini depicting America's Founding Fathers as a diverse group is an interesting case of over-correcting for bias.
There is a very real danger of AI fabricating results and this has already happened. It's relatively easy to get a generative AI tool to cite publications that don't exist.
Furthermore, many AI systems cannot explain why they produce the output they produce.
This is not always a problem. If AI generates a new hypothesis that is then tested by the usual scientific methods, there is no harm done.
However, for some applications a lack of explanation can be a problem.
Replication of results is a basic tenet in science, but if the steps that AI took to reach a conclusion remain opaque, replication and validation become difficult, if not impossible.
And that could harm people's trust in the science produced.
A distinction should be made here between general and narrow AI.
Narrow AI is AI trained to carry out a specific task.
Narrow AI has already made great strides. Google DeepMind's AlphaFold model has revolutionised how scientists predict protein structures.
But there are many other, less well publicised, successes too — such as AI being used at CSIRO to discover new galaxies in the night sky, IBM Research developing AI that rediscovered Kepler's third law of planetary motion, or Samsung AI building AI that was able to reproduce Nobel prize winning scientific breakthroughs.
When it comes to narrow AI applied to science, trust remains high.
AI systems — especially those based on machine learning methods — rarely achieve 100 per cent accuracy on a given task. (In fact, machine learning systems outperform humans on some tasks, and humans outperform AI systems on many tasks. Humans using AI systems generally outperform humans working alone and they also outperform AI working alone. There is a large scientific evidence base for this fact, including this study.) AI working alongside an expert scientist, who confirms and interprets the results, is a perfectly legitimate way of working, and is widely seen as yielding better performance than human scientists or AI systems working alone.
On the other hand, general AI systems are trained to carry out a wide range of tasks, not specific to any domain or use case.
ChatGPT, for example, can create a Shakespearian sonnet, suggest a recipe for dinner, summarise a body of academic literature, or generate a scientific hypothesis.
When it comes to general AI, the problems of hallucinations and bias are most acute and widespread. That doesn't mean general AI isn't useful for scientists — but it needs to be used with care.
This means scientists must understand and assess the risks of using AI in a specific scenario and weigh them against the risks of not doing so.
Scientists are now routinely using general AI systems to help write papers, assist review of academic literature, and even prepare experimental plans.
One danger when it comes to these scientific assistants could arise if the human scientist takes the outputs for granted.
Well-trained, diligent scientists will not do this, of course. But many scientists out there are just trying to survive in a tough industry of publish-or-perish. Scientific fraud is already increasing, even without AI.
AI could lead to new levels of scientific misconduct — either through deliberate misuse of the technology, or through sheer ignorance as scientists don't realise that AI is making things up.
Both narrow and general AI have great potential to advance scientific discovery.
A typical scientific workflow conceptually consists of three phases: understanding what problem to focus on, carrying out experiments related to that problem and exploiting the results as impact in the real world.
AI can help in all three of these phases.
There is a big caveat, however. Current AI tools are not suitable to be used naively out-of-the-box for serious scientific work.
Only if researchers responsibly design, build, and use the next generation of AI tools in support of the scientific method will the public's trust in both AI and science be gained and maintained.
Getting this right is worth it: the possibilities of using AI to transform science are endless.
Google DeepMind's iconic founder Demis Hassabis famously said: ''Building ever more capable and general AI, safely and responsibly, demands that we solve some of the hardest scientific and engineering challenges of our time.'' The reverse conclusion is true as well: solving the hardest scientific challenges of our time demands building ever more capable, safe and responsible general AI.
Australian scientists are working on it. (360info.org) GRS GRS
(This story has not been edited by Devdiscourse staff and is auto-generated from a syndicated feed.)